What is Proteomics?
Scientific Background
Click to expand
Probing Proteins
Almost all diseases manifest themselves as changes in the expression, abundance or signaling status of proteins. Therefore, the precise analysis of the proteome (the entirety of a biological system’s proteins) is a crucial step in the understanding, diagnosis, and treatment of diseases. Mass spectrometry-based proteomics is a powerful technique for the simultaneous analyses of thousands of proteins, fueling biomarker research and drug discovery.
Proteomic Data Analysis
The analysis of proteomic data heavily relies on the automated matching of acquired tandem mass spectra of peptides (fragments of proteins) to protein sequence databases. This process relies on simple assumptions and the key concepts have remained largely unchanged since their introduction in 1993. We believe that we only see the tip of the iceberg. To date, only half of the data acquired from a sample can be identified using classical data analysis workflows, leading to lost productivity, precious samples, and opportunities.
The Power of Deep Learning
Recent developments in the field of machine learning revolutionize all branches of research. Artificial neural networks learn to perform tasks without previously defined rule sets, solely based on annotated training data. We have learned to harness this power to predict properties of peptides like liquid chromatography retention time or fragmentation behavior inside the mass spectrometer.
Predicting Peptide Properties
The MSAID founders developed a generic deep learning framework called INFERYS which learns to predict any peptide property from training data. INFERYS demonstrates superior accuracy performance well above all other current approaches. The algorithm was trained using millions of mass spectra and can be adapted to all common mass spectrometers with minimal additional training. The model is universally applicable to proteins from any organism, creating huge opportunities in areas such as immunopeptidomics, proteogenomics, or metaproteomics. The novel, intelligent search algorithm CHIMERYS is fueled by accurate predictions provided by INFERYS and enables a deeper, more comprehensive data analysis.
Proteomics
Proteins are molecular machines that facilitate a lot of processes necessary to sustain life. They provide a large variety of functions, from structure to metabolism and regulation in every living organism [1]. Proteomics as a field focuses on the identification and quantification of proteins on a large scale. Research questions may be on protein abundance, the variety of proteoforms due to post-translational modifications (PTMs), and stable or transient protein-protein interactions.
Almost all diseases manifest themselves as changes in the expression, abundance or signaling status of proteins. Therefore, the precise analysis of the proteome (the entirety of a biological system’s proteins) is a crucial step in the understanding, diagnosis, and treatment of diseases. Mass spectrometry-based proteomics is a powerful technique for the simultaneous analyses of thousands of proteins, fueling biomarker research and drug discovery.
Fields of Investigation
Proteomics is commonly used to investigate proteins on a large scale:
-
when and where the proteins are expressed in what quantity
-
how proteins are modified by post-translational modifications (PTMs) such as phosphorylation2
-
rates of protein production, degradation, and steady-state abundance
-
how proteins interact with other proteins and protein-complexes [2]
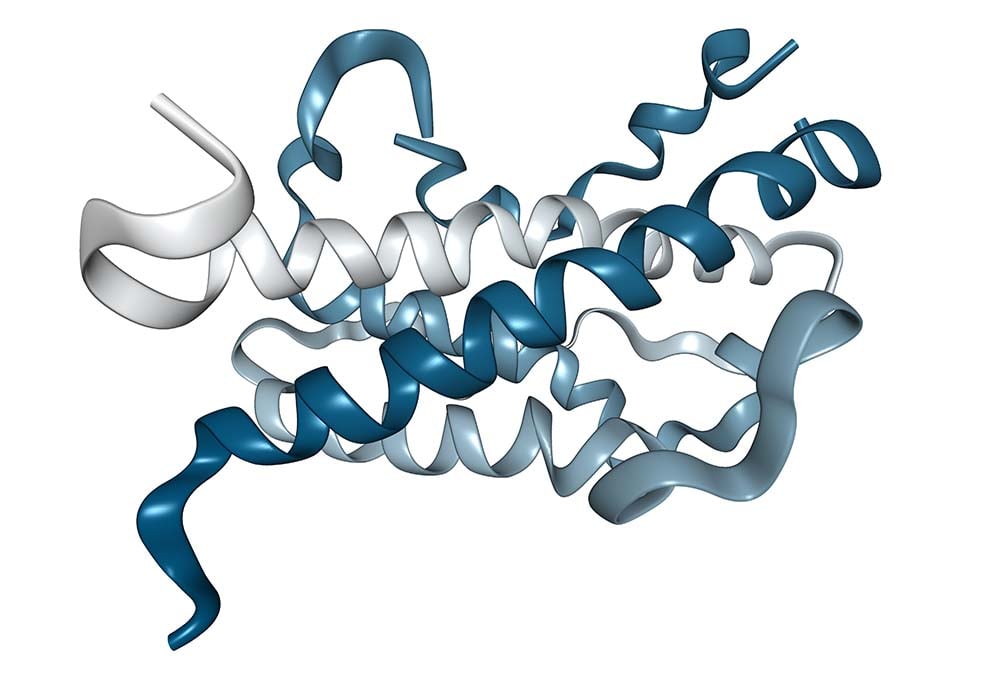
Proteomics aims to be a breakthrough technology that will allow doctors to better diagnose and treat diseases. Large research interest is finding biological markers that signal disease, targets for drugs, and a detailed understanding of biology on the molecular level. [3]
Proteomic Technologies & Workflow
Proteins can be investigated using several technologies. These can be roughly categorized into antibody-based techniques, array-based techniques, and mass spectrometry-based technologies. Mass spectrometry-based proteomics has developed into the workhorse for the large-scale investigation of proteins, largely due to its throughput and independence from biological reagents.
-
Bottom-up proteomics: Technology relying on digesting proteins to peptides using proteolytic enzymes (e.g. Trypsin). This technology has the advantage of being applicable to very complex samples, no prior knowledge of the sample (besides origin) is needed.
-
Liquid chromatography (LC): Technology of separating peptides prior to mass spectrometry. Usually directly interfaced with the mass spectrometer in bottom-up proteomics experiments where peptides are directly ionized and injected in the mass spectrometer after separation.
-
Mass spectrometer (MS): Instruments determining the mass overcharge of peptides by recording mass spectra (lists of masses and corresponding intensities/quantities). Usually, the mass of the intact peptide is determined before fragmenting the peptide in shorter fragments to determine the amino acid sequence.
-
Bioinformatics: Software and databases are enabling technologies for proteomics by automatically assigning acquired mass spectra to peptide sequences. This enables searching hundreds of thousands of spectra in a short period of time in contrast to manually assigning spectra to peptides and therefore proteins. [4]
Proteomics Software
The analysis of proteomic data heavily relies on the automated matching of acquired tandem mass spectra of peptides (fragments of proteins) to protein sequence databases. This process relies on simple assumptions and the key concepts have remained largely unchanged since their introduction in 1993. MSAID replaces current algorithms with powerful, AI-based solutions and paves the way for a deeper, and more reliable way of interrogating proteomics data. Powered by vast amounts of training data, we develop deep learning models for bottom-up proteomics and integrate them into innovative software solutions. We make our software and services easily and readily available, thereby boosting the use of machine learning in the field of proteomics.
INFERYS, INFERYS Rescoring, and CHIMERYS are integrated into Thermo Scientific™ Proteome Discoverer™ 3.0 software. For more information and licensing, please visit thermofisher.com/proteomediscoverer.
References
[1] Graves PR, Haystead TA. Molecular biologist's guide to proteomics. Microbiol Mol Biol Rev. 2002 Mar;66(1):39-63; table of contents.
DOI: 10.1128/MMBR.66.1.39-63.2002. PMID: 11875127; PMCID: PMC120780.
[2] Ramazi S, Zahiri J. Posttranslational modifications in proteins: resources, tools and prediction methods. Database (Oxford). 2021 Apr 7;2021:baab012.
DOI: 10.1093/database/baab012. PMID: 33826699; PMCID: PMC8040245.
[3] Cho WC. Proteomics technologies and challenges. Genomics Proteomics Bioinformatics. 2007 May;5(2):77-85.
DOI: 10.1016/S1672-0229(07)60018-7. PMID: 17893073; PMCID: PMC5054093.
[4]Angel, T. E., Aryal, U. K., Hengel, S. M., Baker, E. S., Kelly, R. T., Robinson, E. W., & Smith, R. D. (2012). Mass spectrometry-based proteomics: Existing capabilities and future directions. Chemical Society Reviews, 41(10), 3912.
DOI: 10.1039/c2cs15331a