What is Artificial Intelligence?
Artificial Intelligence (AI)
Artificial intelligence (AI) is a rapidly growing field that encompasses machine learning, natural language processing, computer vision, and more. It is a branch of computer science that aims to create intelligent machines that can perform tasks that typically require human intelligence, such as understanding natural language, recognizing images, and making decisions.
AI has many advantages, including:
-
Efficiency: AI can process large amounts of data quickly and accurately, which can save time and money.
-
Automation: AI can automate repetitive and mundane tasks, freeing up humans to focus on more important and creative work.
-
Accuracy: AI can be more accurate than humans in certain tasks, such as image and speech recognition.
-
Personalization: AI can be used to create personalized experiences for users, such as personalized product recommendations.
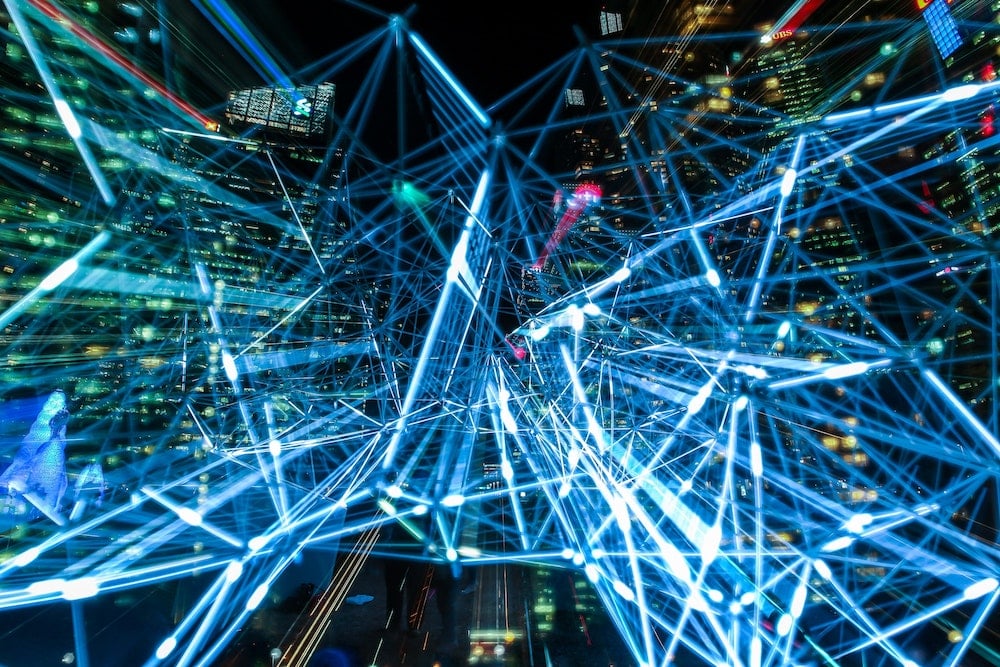
Artificial Intelligence in Proteomic Analysis
-
Protein identification: AI-based algorithms can be used to identify proteins from mass spectrometry data, improving the accuracy and speed of protein identification by finding distinct features that separate correct from incorrect identifications [1,2].
-
Peptide property prediction: In proteomics, peptide properties such as fragmentation or retention time are important clues for protein identification and quantification. Machine learning models can be used to accurately predict those [3-5].
-
Protein-protein interaction prediction: AI-based algorithms can be used to predict potential interactions between proteins, helping researchers understand how proteins function in complex relationships in living organisms [6,7].
-
Post-translational modification analysis: AI-based algorithms can be used more sensitively and more accurately analyze post-translational modifications, which are (transient) chemical modifications that occur on proteins after they are translated from genetic information [8,9].
-
Functional annotation: AI-based algorithms can be used to classify proteins into functional categories, helping researchers understand the roles of different proteins in living organisms [10].
-
Pattern detection: AI-based algorithms can detect complex patterns in biological regulation hidden from the human eye. Such patterns may be indicative of disease states and reveal biomarker signatures to be monitored [11].
Overall, AI is playing an increasingly important role in the field of proteomics, helping researchers to more efficiently and accurately analyze large amounts of data and make new discoveries about the proteins in living organisms.
Artificial Intelligence at MSAID
At MSAID, we understand and harvest the power and potential of artificial intelligence (AI) for the field of proteomics. We are dedicated to pushing the boundaries of what is possible with AI and are constantly exploring new and innovative ways to harness its power to improve our computational analysis of mass spectrometry-based data.
One of our key areas of focus is using deep learning techniques to predict peptide properties for bottom-up proteomics. By incorporating these models into our software solutions, we can provide our clients with powerful tools for data analysis that are both accurate and easy to use. In addition to our deep learning capabilities, we also utilize other branches of AI such as machine learning and tools inspired by natural language processing to improve data analysis pipelines, which gives us a competitive advantage over other companies in the field.
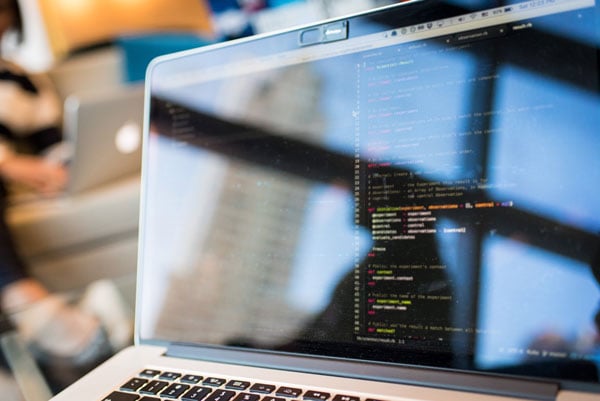
We are committed to making our software and services easily accessible to everyone. That's why we strive to keep our solutions simple to use and easy to understand, making it easy for our clients to gain insights from their data and make better-informed decisions.
We are committed to making our software and services easily accessible to everyone. That's why we strive to keep our solutions simple to use and easy to understand, making it easy for our clients to gain insights from their data and make better-informed decisions.
If you're interested in learning more about how our customized solutions can help you to advance your data analysis pipelines and stay ahead of the competition, please don't hesitate to contact us. We would be happy to answer any questions you may have and help you to find the right solution for your needs.
References
[1] Zolg DP, Gessulat S, Paschke C, Graber M, Rathke-Kuhnert M, Seefried F, Fitzemeier K, Berg F, Lopez-Ferrer D, Horn D, Henrich C, Huhmer A, Delanghe B, Frejno M. INFERYS rescoring: Boosting peptide identifications and scoring confidence of database search results. Rapid Commun Mass Spectrom. 2021 May 20:e9128. DOI: 10.1002/rcm.9128. Epub ahead of print. PMID: 34015160.
[2] Wilhelm M, Zolg DP, Graber M, Gessulat S, Schmidt T, Schnatbaum K, Schwencke-Westphal C, Seifert P, de Andrade Krätzig N, Zerweck J, Knaute T, Bräunlein E, Samaras P, Lautenbacher L, Klaeger S, Wenschuh H, Rad R, Delanghe B, Huhmer A, Carr SA, Clauser KR, Krackhardt AM, Reimer U, Kuster B. Deep learning boosts sensitivity of mass spectrometry-based immunopeptidomics. Nat Commun. 2021 Jun 7;12(1):3346. DOI: 10.1038/s41467-021-23713-9. Erratum in: Nat Commun. 2021 Jun 23;12(1):4002. PMID: 34099720; PMCID: PMC8184761.
[3] Zhou XX, Zeng WF, Chi H, Luo C, Liu C, Zhan J, He SM, Zhang Z. pDeep: Predicting MS/MS Spectra of Peptides with Deep Learning. Anal Chem. 2017 Dec 5;89(23):12690-12697. DOI: 10.1021/acs.analchem.7b02566. Epub 2017 Nov 21. PMID: 29125736.
[4] Gessulat S, Schmidt T, Zolg DP, Samaras P, Schnatbaum K, Zerweck J, Knaute T, Rechenberger J, Delanghe B, Huhmer A, Reimer U, Ehrlich HC, Aiche S, Kuster B, Wilhelm M. Prosit: proteome-wide prediction of peptide tandem mass spectra by deep learning. Nat Methods. 2019 Jun;16(6):509-518.
DOI: 10.1038/s41592-019-0426-7. Epub 2019 May 27. PMID: 31133760.
[5] Tiwary S, Levy R, Gutenbrunner P, Salinas Soto F, Palaniappan KK, Deming L, Berndl M, Brant A, Cimermancic P, Cox J. High-quality MS/MS spectrum prediction for data-dependent and data-independent acquisition data analysis. Nat Methods. 2019 Jun;16(6):519-525.
DOI: 10.1038/s41592-019-0427-6. Epub 2019 May 27. PMID: 31133761
[6] Du X, Sun S, Hu C, Yao Y, Yan Y, Zhang Y. DeepPPI: Boosting Prediction of Protein-Protein Interactions with Deep Neural Networks. J Chem Inf Model. 2017 Jun 26;57(6):1499-1510. DOI: 10.1021/acs.jcim.7b00028. Epub 2017 May 26. PMID: 28514151.
[7] Hashemifar S, Neyshabur B, Khan AA, Xu J. Predicting protein-protein interactions through sequence-based deep learning. Bioinformatics. 2018 Sep 1;34(17):i802-i810. DOI: 10.1093/bioinformatics/bty573. PMID: 30423091; PMCID: PMC6129267.
[8] Luo F, Wang M, Liu Y, Zhao XM, Li A. DeepPhos: prediction of protein phosphorylation sites with deep learning. Bioinformatics. 2019 Aug 15;35(16):2766-2773. DOI: 10.1093/bioinformatics/bty1051. PMID: 30601936; PMCID: PMC6691328.
[9] Zeng WF, Zhou XX, Zhou WJ, Chi H, Zhan J, He SM. MS/MS Spectrum Prediction for Modified Peptides Using pDeep2 Trained by Transfer Learning. Anal Chem. 2019 Aug 6;91(15):9724-9731. DOI: 10.1021/acs.analchem.9b01262. Epub 2019 Jul 8. PMID: 31283184.
[10] Bileschi ML, Belanger D, Bryant DH, Sanderson T, Carter B, Sculley D, Bateman A, DePristo MA, Colwell LJ. Using deep learning to annotate the protein universe. Nat Biotechnol. 2022 Jun;40(6):932-937. DOI: 10.1038/s41587-021-01179-w. Epub 2022 Feb 21. PMID: 35190689.
[11] Mann M, Kumar C, Zeng WF, Strauss MT. Artificial intelligence for proteomics and biomarker discovery. Cell Syst. 2021 Aug 18;12(8):759-770.
DOI: 10.1016/j.cels.2021.06.006. PMID: 34411543.
Created with ChatGPT and revised by MSAID