What is Machine Learning?
Machine Learning
Machine learning is the study and design of self-improving systems that maximize a specified aim. Instead of being a set of instructions written by a human expert, a machine learning system accomplishes its goal through learning from data directly. This is done by statistical models or algorithms such as neural networks. After training, the system needs to be able to generalize what it learned to previously unseen data.
The rapidly expanding discipline of data science overlaps with machine learning. It focuses on understanding the data and its inherent relationships. Those insights can then be used in the design of systems and applications or by experts directly or indirectly to improve machine learning-based systems.
Artificial intelligence is an umbrella term that covers data science, machine learning, and statistics. It also covers heuristic approaches that exhibit “intelligent” behavior. One biologically inspired heuristic would be the ant colony optimization algorithm.
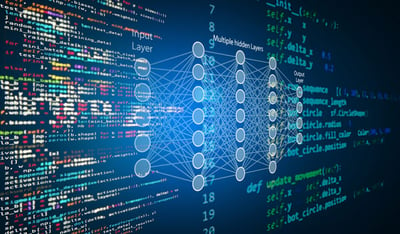
Machine Learning Applications and Proteomics Analysis
Artificial Intelligence at MSAID
As the pioneer in proteomics AI, MSAID replaces outdated algorithms with potent AI-based solutions and paves the way for a more thorough and trustworthy method of querying proteomics data. We use all branches of artificial intelligence to improve the computational analysis of mass spectrometry-based data, whichever fits its purpose best. We facilitate the use of artificial intelligence in the field of proteomics by making our software and services simple to use and easily accessible.
For example, we create deep learning models to predict peptide properties for bottom-up proteomics and incorporate them into creative software solutions, powered by enormous volumes of training data.
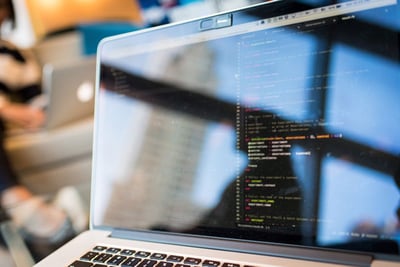
Contact us to learn how our customized solutions can advance your data analysis pipelines and help you to gain an advantage over your competitors.
References
[1] Swan AL, Mobasheri A, Allaway D, Liddell S, Bacardit J. Application of machine learning to proteomics data: classification and biomarker identification in postgenomics biology. OMICS. 2013 Dec;17(12):595-610. DOI: 10.1089/omi.2013.0017. Epub 2013 Oct 12. PMID: 24116388; PMCID: PMC3837439.